EAM in constant evolution
Enterprise Asset Management (EAM) is a constantly evolving field. While specific trends may vary from industry to industry and company to company, here are some general trends in EAM:
IoT (Internet of Things) and connected sensors
Artificial Intelligence (AI) and Machine Learning (ML)
Cloud Computing / SaaS offering
Mobility
Sustainability and risk management
Integration with other systems
It is essential to note that the evolution of EAM trends depends on technological advances, specific industry needs, and changing business requirements. Organizations need to keep abreast of developments to get the most out of their asset management systems.
Partners & Customers
They trust us
Join many supported companies




















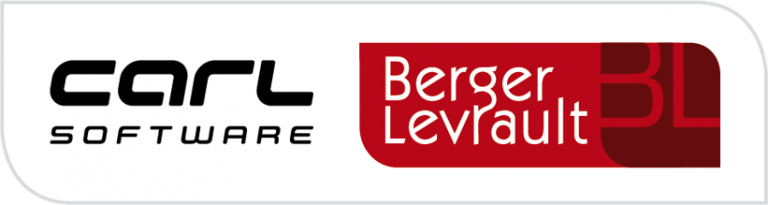
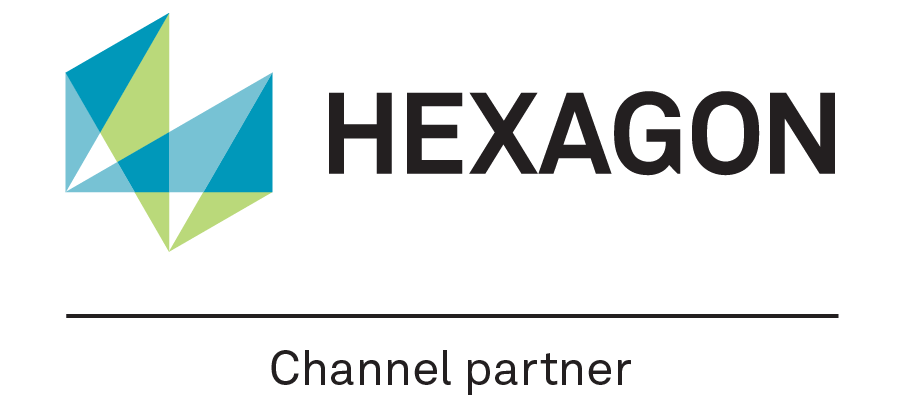
L'impact de la data dans la maintenance prédictive
The impact of data in predictive maintenance Data plays a central role in the development and implementation of predictive maintenance. By exploiting real-time data, companies can anticipate potential failures, plan maintenance more efficiently, and reduce the costs associated with unplanned interruptions.
Some of the major impacts of data in the context of predictive maintenance include, but are not limited to, the following :
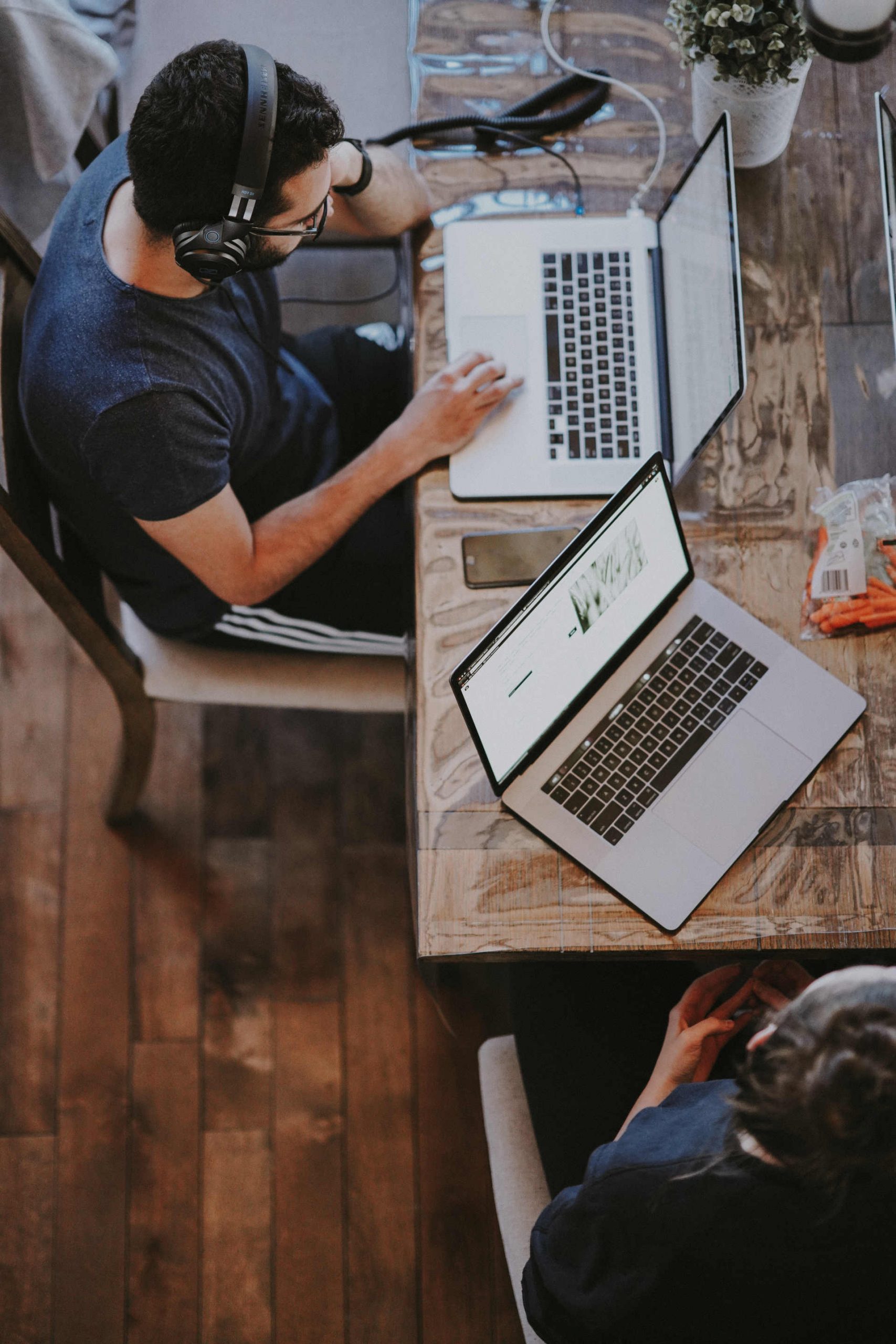
Early detection of anomalies
Predictive analysis using machine learning
Reducing unplanned downtime
Optimizing maintenance activities
Proactive spare parts management
Improving safety
Reducing maintenance costs
Adaptation to actual operating conditions
In conclusion, the impact of data in predictive maintenance is significant, bringing significant improvements in terms of operational efficiency, maintenance costs, and equipment availability.
FAQ - ELAZUR Answers your questions
Choose EAM software by assessing your needs, comparing features, checking usability, and asking other similar companies for recommendations.
The benefits include extended asset life, reduced maintenance costs, better planning, and more efficient management.
EAM solutions enable proactive maintenance planning, efficient spare parts management and extended asset life thanks to preventive maintenance. This reduces long-term maintenance costs.
For all inquiries, Contact us Elazur answers your questions.
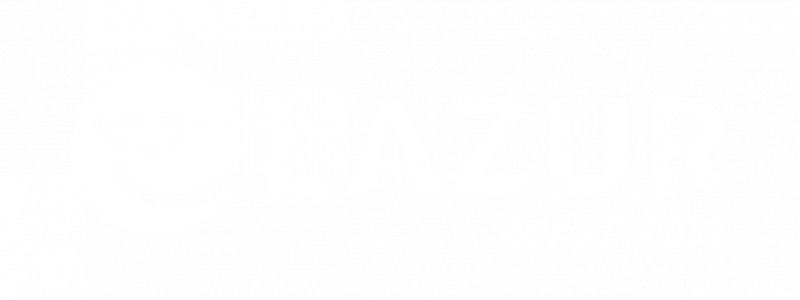